Transforming Businesses Through Data Labeling
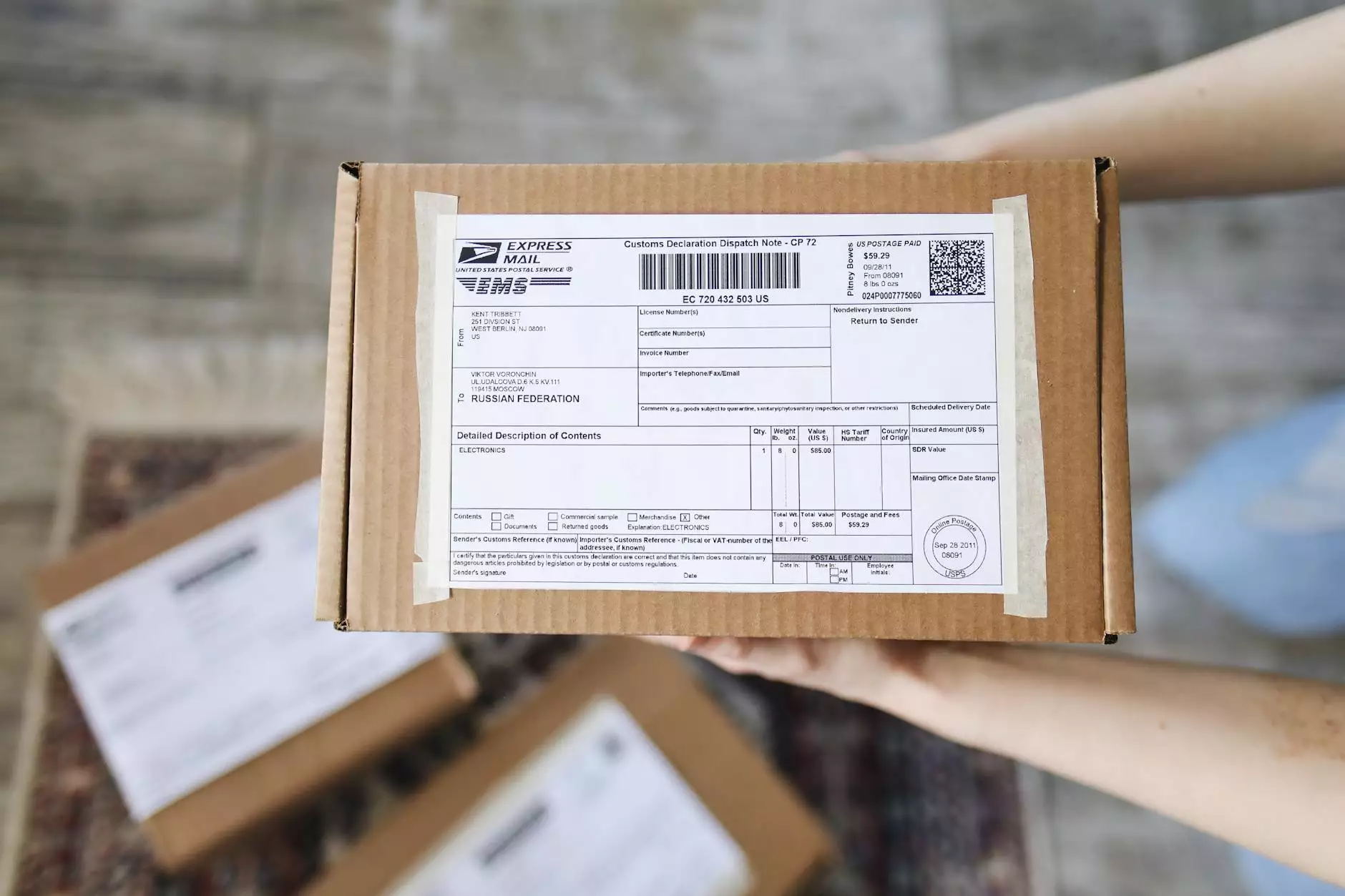
Data labeling is a crucial process in the realm of artificial intelligence (AI) and machine learning (ML) that directly impacts a business's ability to derive actionable insights from data. In an age where data is the new oil, understanding how to effectively label data can set a company apart from its competitors, driving innovation and efficiency. At Keymakr, we recognize the unique value that data labeling brings to software development, enabling businesses to harness the full potential of their data.
The Importance of Data Labeling in Business
Businesses today are inundated with vast amounts of data from various sources. Whether it's customer feedback, transactional data, or unstructured content from social media, the raw data is often not usable in its initial form. This is where data labeling comes into play. It involves the process of annotating or tagging data so that machines can better understand and interpret it.
Key Uses of Data Labeling
- Training Machine Learning Models: A well-labeled dataset is essential for training machine learning models. Models learn from labeled data and improve their accuracy over time.
- Enhancing Customer Experience: Businesses that leverage labeled data can personalize customer experiences based on insights derived from their interactions.
- Efficient Data Categorization: Labeled data allows businesses to categorize and analyze large datasets effectively, facilitating faster decision-making processes.
- Improving Product Development: Understanding customer preferences through labeled data can lead to the development of products that better meet market demands.
The Data Labeling Process
Implementing an effective data labeling strategy involves several steps that ensure high-quality and consistent results. Below is a breakdown of the process:
1. Define Objectives
Before beginning the labeling process, it's vital to define the objectives clearly. What are the goals of the data labeling? Are you looking to develop a facial recognition system, sentiment analysis tool, or an image classification model? Establishing these goals will guide the labeling process.
2. Choose Labeling Techniques
There are several labeling techniques available, such as:
- Manual Labeling: Human annotators manually tag the data, which can ensure accuracy but may be time-consuming.
- Automated Labeling: Machine learning algorithms can assist in labeling data, particularly when dealing with massive datasets.
- Crowdsourcing: Engaging a distributed workforce can speed up the process while maintaining quality through various checks and balances.
3. Select the Right Tools
Numerous tools can facilitate the data labeling process. Choosing the right software is critical. Keymakr offers innovative solutions that streamline this process, providing user-friendly interfaces and robust analytics.
4. Quality Assurance
To ensure that the labeled data is of high quality, implementing a quality assurance (QA) step is essential. This may involve reviewing a sample of labeled data to ensure compliance with the labeling guidelines. Regular audits can help maintain standards.
5. Continuous Improvement
As ML models learn and evolve, the data labeling process should also be revisited. Continuous feedback from the model's performance can highlight areas where the labeling process can be refined or adjusted to improve accuracy and relevance.
Benefits of Data Labeling for Businesses
Investing in data labeling can yield significant benefits for businesses, such as:
1. Increased Accuracy in AI Models
Accurate labeling directly translates to better-performing AI models. Businesses can achieve higher accuracy rates, reducing errors and increasing trust in AI-driven decisions.
2. Faster Time-to-Market
By streamlining the labeling process and employing efficient tools, businesses can design and launch AI applications faster, staying ahead of the competition.
3. Enhanced Data Utilization
Properly labeled data allows businesses to unlock insights that may have otherwise remained hidden in raw data, driving strategic initiatives and operational improvements.
4. Competitive Edge
In a competitive landscape, businesses that effectively leverage labeled data can innovate faster and respond to market changes more adeptly, providing a significant advantage over rivals.
Challenges in Data Labeling
While the benefits are vast, the data labeling process is not without its challenges. Some of the common issues faced by businesses include:
1. Scale and Volume
The sheer volume of data generated by businesses can make labeling a daunting task. Ensuring that large datasets are accurately labeled requires substantial resources.
2. Subjectivity in Labeling
In cases where human judgment is required, there may be inconsistencies in labeling due to subjective interpretations. This can compromise the quality of the labeled dataset.
3. Evolving Use-Cases
As technologies evolve, so do the requirements for data labeling. Businesses need to remain agile and adapt their labeling strategies to align with new model requirements and market demands.
Best Practices for Effective Data Labeling
To overcome the challenges associated with data labeling, businesses can adopt best practices, such as:
- Establish Clear Guidelines: Define clear and comprehensive guidelines for data labeling to minimize subjectivity and ensure consistency.
- Invest in Training: Regular training for annotators can enhance their skills and understanding of the requirements, resulting in better-labeled data.
- Utilize Technology: Leverage advanced labeling tools and AI-assisted labeling processes to increase efficiency and accuracy.
- Run Pilot Projects: Before scaling labeling efforts, conduct pilot projects to test and refine the labeling process.
Case Studies: Success Stories with Data Labeling
Real-world examples can provide insight into the transformative power of data labeling. Consider the following case studies:
1. Healthcare Applications
A healthcare company implemented a machine learning model to predict patient outcomes. They used labeled datasets from past patient records to train their model, achieving an accuracy rate of over 90%. This process significantly improved patient care and resource allocation within their facilities.
2. E-Commerce Personalization
An e-commerce giant utilized labeled data to categorize customer reviews. By understanding sentiments associated with different products, they personalized recommendations for users, leading to a 30% increase in sales conversions.
3. Autonomous Vehicles
In the automotive industry, manufacturers rely heavily on labeled image datasets to train their autonomous driving systems. Accurate labeling of road signs, pedestrians, and obstacles has enhanced safety and efficiency, accelerating the development of self-driving technologies.
The Future of Data Labeling in Business
As industries continue to evolve, the role of data labeling will only increase in significance. Emerging technologies such as natural language processing (NLP), computer vision, and advanced robotics will demand even more precise and comprehensive labeled data. Businesses that proactively invest in refining their data labeling processes will position themselves as leaders in their respective fields.
At Keymakr, we believe that by embracing the complexities of data labeling, businesses can unlock unprecedented opportunities for growth and innovation. By prioritizing quality, accuracy, and efficiency in data labeling, companies can ensure their AI and machine learning initiatives lead to meaningful outcomes that drive their success.
Conclusion
In conclusion, the significance of data labeling in the modern business landscape cannot be overstated. Whether it's enhancing customer experiences, improving operational efficiency, or driving innovation, proper data labeling is essential. By investing in high-quality labeling processes, utilizing advanced tools, and adhering to best practices, businesses can harness the full potential of their data, leading to improved outcomes and sustained growth. At Keymakr, we are dedicated to helping businesses navigate the complexities of data labeling, ensuring they achieve their strategic goals through informed decision-making and innovative solutions.