Labeling Tools for Machine Learning: A Comprehensive Guide
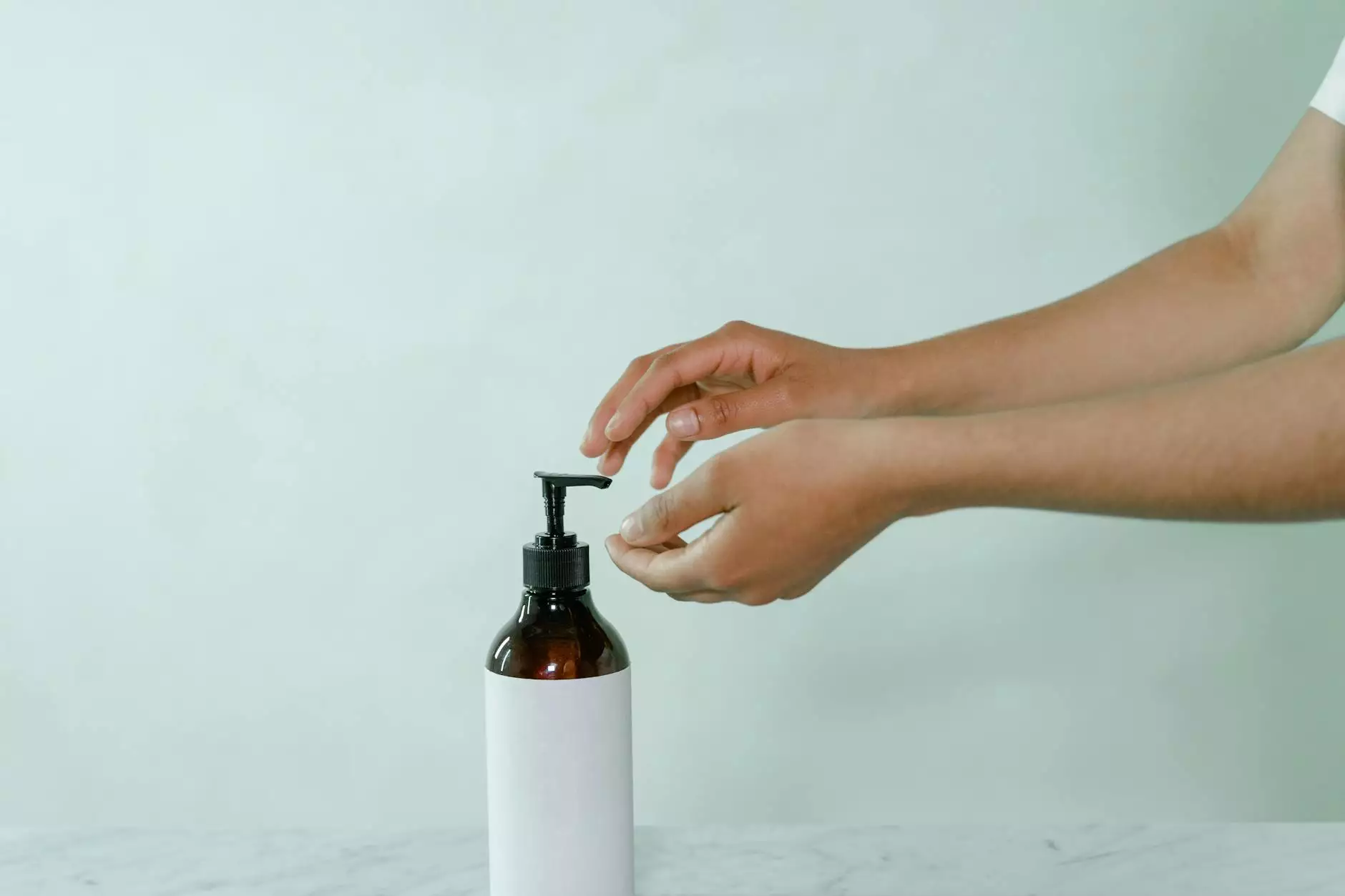
In today’s data-driven world, harnessing the power of machine learning is pivotal for any business aiming to innovate and remain competitive. An essential component of successful machine learning models is the reliance on high-quality labeled data. Consequently, the need for effective labeling tools for machine learning has never been more critical. This article delves into the significance of labeling tools, their functionalities, and the best practices to utilize them for optimal results.
Understanding the Importance of Data Labeling
Data labeling involves the process of annotating data, which is fundamental for supervised learning algorithms. Data can come in various forms including text, images, audio, and video. Here are some key points highlighting the necessity of data labeling:
- Improves Model Accuracy: Well-labeled data dramatically increases the accuracy of machine learning models.
- Facilitates Model Training: Supervised learning can only occur with labeled data, making it vital for training reliable models.
- Enhances Data Interpretability: Labeling helps in understanding the data better and makes it easier to derive insights.
Key Features of Effective Labeling Tools
When selecting labeling tools for machine learning, there are several features that one should look for. Here’s a breakdown:
- User-Friendly Interface: The tool should be easy to navigate and use, allowing for both novice and experienced users to label data efficiently.
- Support for Multiple Data Types: It should accommodate different formats such as images, text, and audio clips.
- Collaboration Features: The ability to work collaboratively with team members can improve workflow.
- Automation Capabilities: Advanced tools may offer automation to streamline repetitive tasks, enhancing overall productivity.
- Quality Control Mechanisms: Features that allow for checking the quality and consistency of labeled data are essential.
Best Labeling Tools for Machine Learning
There is a plethora of labeling tools available in the market today. Here’s a list of some of the most effective options:
1. Labelbox
Labelbox is a premier tool offering a rich set of features tailored for various data types. It provides:
- A collaborative interface that allows teams to work together in real-time.
- Customizable workflows for different projects.
- Integrations with popular cloud services for seamless data management.
2. Supervisely
Supervisely is particularly well-suited for image and video annotation. Key features include:
- A comprehensive set of annotations types like bounding boxes and polygons.
- Powerful tools for 3D and interactive annotation.
- Data management functionalities that simplify handling large datasets.
3. Snorkel
Snorkel specializes in programmatically generating labeled data and is ideal for organizations with limited labeled data. Benefits include:
- A focus on weak supervision, allowing for blending multiple labeling functions.
- Scalability to handle large datasets without explicit manual labeling.
4. VGG Image Annotator (VIA)
The VGG Image Annotator is a free tool suitable for those on a budget. Highlighted features are:
- Lightweight interface for quick labeling tasks.
- Support for various annotation types including bounding boxes, regions, and points.
- Self-contained, requiring no internet connection.
5. Prodigy
Prodigy is an annotation tool that utilizes active learning to improve labeling efficiency. Its strengths include:
- Interactive workflows that adapt based on user feedback.
- Ability to integrate with existing machine learning models for better suggestions.
Best Practices for Effective Data Labeling
To maximize the benefits of using labeling tools for machine learning, adhere to these best practices:
- Define Clear Annotation Guidelines: Having clear and concise guidelines will ensure consistency across data labeling tasks.
- Utilize Active Learning: Combine human effort with machine intelligence by using models to prioritize data for labeling.
- Incorporate Quality Checks: Regularly review labeled data to identify inconsistencies and retrain annotators as needed.
- Engage Collaboratively: Foster communication among team members to share insights and improve the labeling process.
Conclusion: The Future of Labeling Tools in Machine Learning
The importance of labeling tools for machine learning cannot be overstated. As we move towards more advanced machine learning applications, the complexity and volume of data will require even more sophisticated labeling solutions. Businesses like KeyMakr not only provide critical services in the realm of keys and locksmithing but can also leverage machine learning through effective data labeling to enhance their operational efficiencies.
As organizations recognize the undeniable link between high-quality labeled data and machine learning success, investing in the right tools becomes imperative. The future is promising, and with the right approaches, businesses can harness the full potential of machine learning, ensuring they stay ahead in their respective industries.
For more information and resources on labeling tools for machine learning, feel free to contact us or visit our website!